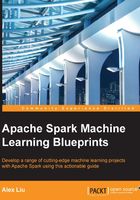
Accessing and loading datasets
In this section, we will review some publicly available datasets and cover methods of loading some of these datasets into Spark. Then, we will review several methods of exploring and visualizing these datasets on Spark.
After this section, we will be able to find some datasets to use, load them into Spark, and then start to explore and visualize this data.
Accessing publicly available datasets
As there is an open source movement to make software free, there is also a very active open data movement that made a lot of datasets freely accessible to every researcher and analyst. At a worldwide scale, most governments make their collected datasets open to the public. For example, on http://www.data.gov/, there are more than 140,000 datasets available to be used freely, which are spread over agriculture, finance, and education.
Besides open data coming from various governmental organizations, many research institutions also collect a lot of very useful datasets and make them available for public use. For our use in this book, the following is a list:
- A very rich click dataset is provided by University of Indiana with 53.5 billion HTTP requests. To access this data, go to http://cnets.indiana.edu/groups/nan/webtraffic/click-dataset/.
- The well known UC Irvine Machine Learning Repository archive offers more than 300 datasets for exploration. To access their datasets, go to https://archive.ics.uci.edu/ml/datasets.html.
- Project Tycho® at University of Pittsburgh provides data from all weekly notifiable disease reports in the United States dating back to 1888. To access their datasets, go to http://www.tycho.pitt.edu/.
- ICPSR has many datasets that are not very big but are of good quality for research. To access their data, go to http://www.icpsr.umich.edu/index.html.
- The airline performance data from 1987 to 2008 is a well-known dataset and is also very big, with 120 million records, as it has been used in many researches and a few competitions. To access this data, go to http://stat-computing.org/dataexpo/2009/the-data.html.
Loading datasets into Spark
There are many ways of loading datasets into Spark or directly connecting to a data source for Spark. As Apache Spark develops with new releases once every three weeks, newer and easier methods are expected to become available to users as well as ways of representing imported data.
For example, JdbcRDD
was the preferred way to connect with a relational data source and transfer data elements to RDD up until Spark 1.3. However, from Spark 1.4 onwards, there is an built in Spark datasource API available to connect to a JDBC source using DataFrames.
Loading data is not a simple task as it often involves converting or parsing raw data and dealing with data format transformation. The Spark datasource API allows users to use libraries based on the Data Source API to read and write dataframes between various formats from various systems. Also Spark's datasource API's data access is very efficient as it is powered by the Spark SQL query optimizer.
To load datasets in as a DataFrame, it is best to use sqlContext.load
, for which we need to specify the following:
- Data source name: This is the source that we load from
- Options: These are parameters for a specific data source—for example, the path of data
For example, we can use the following code:
df1 = sqlContext.read \ . format("json") \ data format is json . option("samplingRatio", "0.01") \ set sampling ratio as 1% . load("/home/alex/data1,json") \ specify data name and location
To export data sets, users can use dataframe.save
or df.write
to save the processed DataFrame to a source; for this, we need to specify the following:
- Data source name: The source that we are saving to
- Save mode: This is what we should do when the data already exists
- Options: These are the parameters for a specific data source—for example, the path of data
The creatExternalTable
and SaveAsTable
commands are also very useful.
Exploring and visualizing datasets
Within Apache Spark, there are many ways to conduct some initial exploration and visualization of the datasets loaded with various tools. Users may use Scala or Python direct with Spark shells. Alternatively, users can take a notebook approach, which is to use the R or Python notebook in a Spark environment, similar to that of DataBricks Workspace. Another option is to utilize Spark's MLlib.
Alternatively, users can directly use Spark SQL and its associated libraries, such as the popular Panda library, for some simple data exploration.
If datasets are already transformed into Spark DataFrames, users may use df.describe().show()
to obtain some simple statistics with values of total count of cases, mean, standard deviation, min, and max for all the columns (variables).
If DataFrame has a lot of columns, users should specify columns in df.describe(column1, column2, …).show()
to just obtain simple descriptive statistics of the columns they are interested in. You may also just use this command to select the statistics you need:
df.select([mean('column1'),min('column1'),max('column1')]).show()
Beyond this, some commonly used commands for covariance, correlation, and cross-tabulation tables are as follows:
df.stat.cov('column1', 'column2') df.stat.corr('column1', 'column2') df.stat.crosstab("column1", "column2").show()
If using the DataBricks workspace, users can create an R notebook; then they will be back to the familiar R environment with access to all the R packages and can take advantage of the notebook approach for an interactive exploration and visualization of datasets. Take a look at the following:
> summary(x1) Min. 1st Qu. Median Mean 3rd Qu. Max. 0.000 0.600 2.000 2.667 4.000 8.000 > plot(x1)

As we will start using the DataBricks Workspace a lot from now on, it is recommended for users to sign up at https://accounts.cloud.databricks.com/registration.html#signup for a trial test. Go to its main menu to its upper-left corner and set up some clusters, as follows:

Then, users can go to the same main menu, click on the down arrow on the right-hand side of Workspace and navigate to Create | New Notebook as follows to create a notebook:

When the Create Notebook dialog appears, you need to perform the following:
- Enter a unique name for your notebook
- For language, click on the drop-down menu and select R
- For cluster, click on the drop–down menu and select the cluster you previously created