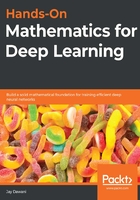
Cholesky decomposition
As I'm sure you've figured out by now, there is more than one way to factorize a matrix, and there are special methods for special matrices.
The Cholesky decomposition is square root-like and works only on symmetric positive definite matrices.
This works by factorizing A into the form LLT. Here, L, as before, is a lower triangular matrix.
Do develop some intuition. It looks like this:

However, here, L is called a Cholesky factor.
Let's take a look at the case where .
We know from the preceding matrix that ; therefore, we have the following:

Let's multiply the upper and lower triangular matrices on the right, as follows:

Writing out A fully and equating it to our preceding matrix gives us the following:

We can then compare, element-wise, the corresponding entries of A and LLT and solve algebraically for , as follows:

We can repeat this process for any symmetric positive definite matrix, and compute the li,j values given ai,j.