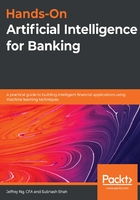
Applications of AI in banking
According to theMcKinsey Global Institute (https://www.mckinsey.com/~/media/mckinsey/industries/advanced%20electronics/our%20insights/how%20artificial%20intelligence%20can%20deliver%20real%20value%20to%20companies/mgi-artificial-intelligence-discussion-paper.ashx), outof 13 industries, financial services ranked third in AI adoption, followed by the high-tech, telecommunications, and automotive and assembly industries.
As theMckinsey report does not mention the use case in banking, with a bit of research, perhaps we can take a look at the four ways in which AI creates values, as shown in the following list:
- Project: Forecast and anticipate demand, improve sourcing, and reduce inventory (capital).
- Produce: Provide services at a lower cost or higher quality.
- Promote: Provide offers for the right price with the right message for the right customers at the right time.
- Provide: Rich, personal, and convenient user experiences.
Let's examine how each finance participant applies AI to the following aspects, as shown in the following table:

Across the board, we can now see how data is being leveraged for smart decision making in the field of finance: more data points and a higher speed of exchange can lead to a much lower cost of finance. More detailed examples will be provided in the following chapters.
How do we attain this lower cost? Essentially, we get it by having fewer hours spent working on producing an aspect of the banking service.
Impact of AI on a bank's profitability
To give you an idea of AI's impact on a bank's profitability, let's take a look at some simple estimates from two perspectives: the improvement of model accuracy and the time spent to run/train the model.
Over the past 10 years, the clock rate and the number of cores have improved tenfold, from around 300 cores to around 3,000 cores.
I have compared the shallow machine learning or statistical model I experienced a decade ago to what I see today with deep neural networks. The model accuracy of neural networks improves the model from around 80% to over 90%, with a 12.5% rate of improvement. The following table shows improvements in the memory data rate, bus width, and size:

If the cost-to-income ratio is around 70% for banks, then the automation rate will likely reduce the ratios by half to 35%. However, the cost of technology investment will take up another 5-10%, taking the target cost-to-income ratios from 40% to 45% following the proliferation of AI in banking. This will impact the banks in developed countries more, as the cost of labor is quite high compared to emerging markets.
Improving the accuracy of forecasts will reduce the cost of the bank's forecast ability further, which, in turn, will reduce the cost of risk by 15%. My personal view is that, for developed countries, the cost of risk is at 50 basis points (bps) of the overall asset; a reduction of 15% on the bank's cost of risk cannot have a significant impact on their profitability.
The improvement in forecast accuracy and convenience will improve the accessibility of banks, which means they can reach a bigger market that was not considered feasible in the past. That is, the profitability ratio of return on the equity does not reflect the impact; rather, it will be shown in the size of the bank and the market capitalization of banks. It should generate an improvement of 15% given a wider reach.
All in all, there is an improvement in return by 80%, from 7.9% Return on Equity (ROE) to 14.5%. However, there will be additional capital requirements for systemically important banks from 11% to 12%, gradually, which will drag the overall ROE down to13.3%in the target AI adaption stage, with all regulations settling in.